How to get the count of free/used processes/threads in a process Pool
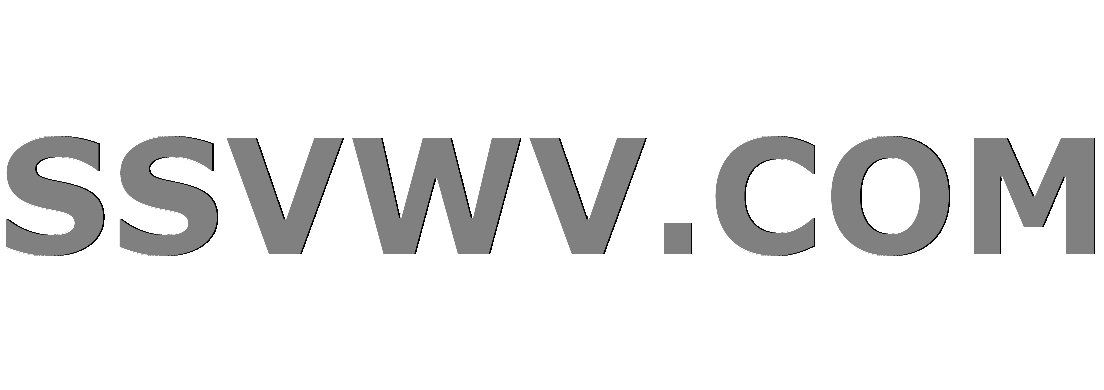
Multi tool use
We can send tasks to a process Pool
.
Is there an easy way to ask the Pool
how many of its workers are currently active with tasks?
I don't want to add a new task if all the processes are currently busy.
Perhaps I have to track this 'manually', by tracking calling .ready()
on the results from apply_async
in order to see which of the existing tasks have already completed. But I hope there is something simpler?
python multiprocessing
add a comment |
We can send tasks to a process Pool
.
Is there an easy way to ask the Pool
how many of its workers are currently active with tasks?
I don't want to add a new task if all the processes are currently busy.
Perhaps I have to track this 'manually', by tracking calling .ready()
on the results from apply_async
in order to see which of the existing tasks have already completed. But I hope there is something simpler?
python multiprocessing
add a comment |
We can send tasks to a process Pool
.
Is there an easy way to ask the Pool
how many of its workers are currently active with tasks?
I don't want to add a new task if all the processes are currently busy.
Perhaps I have to track this 'manually', by tracking calling .ready()
on the results from apply_async
in order to see which of the existing tasks have already completed. But I hope there is something simpler?
python multiprocessing
We can send tasks to a process Pool
.
Is there an easy way to ask the Pool
how many of its workers are currently active with tasks?
I don't want to add a new task if all the processes are currently busy.
Perhaps I have to track this 'manually', by tracking calling .ready()
on the results from apply_async
in order to see which of the existing tasks have already completed. But I hope there is something simpler?
python multiprocessing
python multiprocessing
asked Nov 20 at 6:34


Aaron McDaid
19k54875
19k54875
add a comment |
add a comment |
1 Answer
1
active
oldest
votes
There's no build-in way if that's your question. A worker idling just blocks at task = get()
. get()
here is the corresponding method of either a multiprocessing.SimpleQueue
, in case your pool is a process-pool, or of a queue.Queue
, in case you use multiprocessing.dummy.Pool
aka multiprocessing.pool.ThreadPool
. There's no internal bookkeeping about the number of idling workers, it's just .get()
and .put()
on queues what triggers distribution of tasks and fetching of results.
You can however, get the count of outstanding tasks when you check the length of the internal pool._cache
. After a processed tasks returns from a worker, the entry (ApplyResult
-instance) there gets deleted. So when you evaluate max(pool._processes - len(pool._cache), 0)
you would get a number of idling workers. The actual value is not reliable, though. Pool
uses three threads for managing its internals...
I built an example which didn't break in testing, but I don't consider it safe to use, although the only danger here would be that a task might be submitted too early. It would also include some time.sleep()
to prevent busy-waiting, but that's always inefficient, because it doesn't (just) unblock as soon there is actually something to do.
Instead, if you insist on using multiprocessing.Pool
, you could do something like the example below shows. I'm using a collections.deque
here as a structure for tasks and results to append()
on the right end and popping from the left end. deque
is optimized for such operations and also used to implement queue.Queue
.
from datetime import datetime
from collections import deque
from multiprocessing import Pool
def busy_foo(x):
for _ in range(int(x)):
1 - 1
print(x)
return x
if __name__ == '__main__':
N_WORKERS = 4
tasks = deque(
[(busy_foo, (i,)) for i in range(int(150e6), int(150e6 + 10))]
)
pool = Pool(N_WORKERS)
print(f'{datetime.now()}: Initial distribution of {N_WORKERS} tasks.')
async_results = deque(
[pool.apply_async(*tasks.popleft()) for _ in range(N_WORKERS)]
)
fetched_results =
while tasks: # while still undistributed (non-popped) tasks left
print(f'{datetime.now()}: Waiting for async_result.')
# `.get()` blocks until result available
fetched_results.append(async_results.popleft().get())
print(f'{datetime.now()}: Submitting next task.')
async_results.append(pool.apply_async(*tasks.popleft()))
print(f'{datetime.now()}: All tasks submitted.')
while async_results: # get rest of outstanding results
print(f'{datetime.now()}: Waiting for async_result.')
fetched_results.append(async_results.popleft().get())
pool.close()
pool.join()
print('n', fetched_results)
What happens is that you, in a first round, distribute as much tasks as much workers you have in your pool. Then you while-loop over the remaining tasks and block-wait on the async-results. Whenever a result is finished, you pop the next task from the tasks-deque and submit it into the pool. When all tasks are submitted, you just await the remaining async_results in a second loop.
Output from this example looks like this:
2018-11-20 20:21:55.430073: Initial distribution of 4 tasks.
2018-11-20 20:21:55.430166: Waiting for async_result.
150000000
2018-11-20 20:22:12.306330: Submitting next task.
2018-11-20 20:22:12.306414: Waiting for async_result.
150000002
150000003
150000001
2018-11-20 20:22:17.715737: Submitting next task.
2018-11-20 20:22:17.715876: Waiting for async_result.
2018-11-20 20:22:17.715922: Submitting next task.
2018-11-20 20:22:17.726139: Waiting for async_result.
2018-11-20 20:22:17.726201: Submitting next task.
2018-11-20 20:22:17.726263: Waiting for async_result.
150000004
2018-11-20 20:22:32.358946: Submitting next task.
2018-11-20 20:22:32.359040: Waiting for async_result.
150000007
150000005
2018-11-20 20:22:37.984947: Submitting next task.
2018-11-20 20:22:37.990142: All tasks submitted.
2018-11-20 20:22:37.990173: Waiting for async_result.
150000006
2018-11-20 20:22:38.783913: Waiting for async_result.
2018-11-20 20:22:38.783988: Waiting for async_result.
150000008
2018-11-20 20:22:46.364376: Waiting for async_result.
150000009
[150000000, 150000001, 150000002, 150000003, 150000004, 150000005, 150000006, 150000007, 150000008, 150000009]
Process finished with exit code 0
add a comment |
Your Answer
StackExchange.ifUsing("editor", function () {
StackExchange.using("externalEditor", function () {
StackExchange.using("snippets", function () {
StackExchange.snippets.init();
});
});
}, "code-snippets");
StackExchange.ready(function() {
var channelOptions = {
tags: "".split(" "),
id: "1"
};
initTagRenderer("".split(" "), "".split(" "), channelOptions);
StackExchange.using("externalEditor", function() {
// Have to fire editor after snippets, if snippets enabled
if (StackExchange.settings.snippets.snippetsEnabled) {
StackExchange.using("snippets", function() {
createEditor();
});
}
else {
createEditor();
}
});
function createEditor() {
StackExchange.prepareEditor({
heartbeatType: 'answer',
autoActivateHeartbeat: false,
convertImagesToLinks: true,
noModals: true,
showLowRepImageUploadWarning: true,
reputationToPostImages: 10,
bindNavPrevention: true,
postfix: "",
imageUploader: {
brandingHtml: "Powered by u003ca class="icon-imgur-white" href="https://imgur.com/"u003eu003c/au003e",
contentPolicyHtml: "User contributions licensed under u003ca href="https://creativecommons.org/licenses/by-sa/3.0/"u003ecc by-sa 3.0 with attribution requiredu003c/au003e u003ca href="https://stackoverflow.com/legal/content-policy"u003e(content policy)u003c/au003e",
allowUrls: true
},
onDemand: true,
discardSelector: ".discard-answer"
,immediatelyShowMarkdownHelp:true
});
}
});
Sign up or log in
StackExchange.ready(function () {
StackExchange.helpers.onClickDraftSave('#login-link');
});
Sign up using Google
Sign up using Facebook
Sign up using Email and Password
Post as a guest
Required, but never shown
StackExchange.ready(
function () {
StackExchange.openid.initPostLogin('.new-post-login', 'https%3a%2f%2fstackoverflow.com%2fquestions%2f53387493%2fhow-to-get-the-count-of-free-used-processes-threads-in-a-process-pool%23new-answer', 'question_page');
}
);
Post as a guest
Required, but never shown
1 Answer
1
active
oldest
votes
1 Answer
1
active
oldest
votes
active
oldest
votes
active
oldest
votes
There's no build-in way if that's your question. A worker idling just blocks at task = get()
. get()
here is the corresponding method of either a multiprocessing.SimpleQueue
, in case your pool is a process-pool, or of a queue.Queue
, in case you use multiprocessing.dummy.Pool
aka multiprocessing.pool.ThreadPool
. There's no internal bookkeeping about the number of idling workers, it's just .get()
and .put()
on queues what triggers distribution of tasks and fetching of results.
You can however, get the count of outstanding tasks when you check the length of the internal pool._cache
. After a processed tasks returns from a worker, the entry (ApplyResult
-instance) there gets deleted. So when you evaluate max(pool._processes - len(pool._cache), 0)
you would get a number of idling workers. The actual value is not reliable, though. Pool
uses three threads for managing its internals...
I built an example which didn't break in testing, but I don't consider it safe to use, although the only danger here would be that a task might be submitted too early. It would also include some time.sleep()
to prevent busy-waiting, but that's always inefficient, because it doesn't (just) unblock as soon there is actually something to do.
Instead, if you insist on using multiprocessing.Pool
, you could do something like the example below shows. I'm using a collections.deque
here as a structure for tasks and results to append()
on the right end and popping from the left end. deque
is optimized for such operations and also used to implement queue.Queue
.
from datetime import datetime
from collections import deque
from multiprocessing import Pool
def busy_foo(x):
for _ in range(int(x)):
1 - 1
print(x)
return x
if __name__ == '__main__':
N_WORKERS = 4
tasks = deque(
[(busy_foo, (i,)) for i in range(int(150e6), int(150e6 + 10))]
)
pool = Pool(N_WORKERS)
print(f'{datetime.now()}: Initial distribution of {N_WORKERS} tasks.')
async_results = deque(
[pool.apply_async(*tasks.popleft()) for _ in range(N_WORKERS)]
)
fetched_results =
while tasks: # while still undistributed (non-popped) tasks left
print(f'{datetime.now()}: Waiting for async_result.')
# `.get()` blocks until result available
fetched_results.append(async_results.popleft().get())
print(f'{datetime.now()}: Submitting next task.')
async_results.append(pool.apply_async(*tasks.popleft()))
print(f'{datetime.now()}: All tasks submitted.')
while async_results: # get rest of outstanding results
print(f'{datetime.now()}: Waiting for async_result.')
fetched_results.append(async_results.popleft().get())
pool.close()
pool.join()
print('n', fetched_results)
What happens is that you, in a first round, distribute as much tasks as much workers you have in your pool. Then you while-loop over the remaining tasks and block-wait on the async-results. Whenever a result is finished, you pop the next task from the tasks-deque and submit it into the pool. When all tasks are submitted, you just await the remaining async_results in a second loop.
Output from this example looks like this:
2018-11-20 20:21:55.430073: Initial distribution of 4 tasks.
2018-11-20 20:21:55.430166: Waiting for async_result.
150000000
2018-11-20 20:22:12.306330: Submitting next task.
2018-11-20 20:22:12.306414: Waiting for async_result.
150000002
150000003
150000001
2018-11-20 20:22:17.715737: Submitting next task.
2018-11-20 20:22:17.715876: Waiting for async_result.
2018-11-20 20:22:17.715922: Submitting next task.
2018-11-20 20:22:17.726139: Waiting for async_result.
2018-11-20 20:22:17.726201: Submitting next task.
2018-11-20 20:22:17.726263: Waiting for async_result.
150000004
2018-11-20 20:22:32.358946: Submitting next task.
2018-11-20 20:22:32.359040: Waiting for async_result.
150000007
150000005
2018-11-20 20:22:37.984947: Submitting next task.
2018-11-20 20:22:37.990142: All tasks submitted.
2018-11-20 20:22:37.990173: Waiting for async_result.
150000006
2018-11-20 20:22:38.783913: Waiting for async_result.
2018-11-20 20:22:38.783988: Waiting for async_result.
150000008
2018-11-20 20:22:46.364376: Waiting for async_result.
150000009
[150000000, 150000001, 150000002, 150000003, 150000004, 150000005, 150000006, 150000007, 150000008, 150000009]
Process finished with exit code 0
add a comment |
There's no build-in way if that's your question. A worker idling just blocks at task = get()
. get()
here is the corresponding method of either a multiprocessing.SimpleQueue
, in case your pool is a process-pool, or of a queue.Queue
, in case you use multiprocessing.dummy.Pool
aka multiprocessing.pool.ThreadPool
. There's no internal bookkeeping about the number of idling workers, it's just .get()
and .put()
on queues what triggers distribution of tasks and fetching of results.
You can however, get the count of outstanding tasks when you check the length of the internal pool._cache
. After a processed tasks returns from a worker, the entry (ApplyResult
-instance) there gets deleted. So when you evaluate max(pool._processes - len(pool._cache), 0)
you would get a number of idling workers. The actual value is not reliable, though. Pool
uses three threads for managing its internals...
I built an example which didn't break in testing, but I don't consider it safe to use, although the only danger here would be that a task might be submitted too early. It would also include some time.sleep()
to prevent busy-waiting, but that's always inefficient, because it doesn't (just) unblock as soon there is actually something to do.
Instead, if you insist on using multiprocessing.Pool
, you could do something like the example below shows. I'm using a collections.deque
here as a structure for tasks and results to append()
on the right end and popping from the left end. deque
is optimized for such operations and also used to implement queue.Queue
.
from datetime import datetime
from collections import deque
from multiprocessing import Pool
def busy_foo(x):
for _ in range(int(x)):
1 - 1
print(x)
return x
if __name__ == '__main__':
N_WORKERS = 4
tasks = deque(
[(busy_foo, (i,)) for i in range(int(150e6), int(150e6 + 10))]
)
pool = Pool(N_WORKERS)
print(f'{datetime.now()}: Initial distribution of {N_WORKERS} tasks.')
async_results = deque(
[pool.apply_async(*tasks.popleft()) for _ in range(N_WORKERS)]
)
fetched_results =
while tasks: # while still undistributed (non-popped) tasks left
print(f'{datetime.now()}: Waiting for async_result.')
# `.get()` blocks until result available
fetched_results.append(async_results.popleft().get())
print(f'{datetime.now()}: Submitting next task.')
async_results.append(pool.apply_async(*tasks.popleft()))
print(f'{datetime.now()}: All tasks submitted.')
while async_results: # get rest of outstanding results
print(f'{datetime.now()}: Waiting for async_result.')
fetched_results.append(async_results.popleft().get())
pool.close()
pool.join()
print('n', fetched_results)
What happens is that you, in a first round, distribute as much tasks as much workers you have in your pool. Then you while-loop over the remaining tasks and block-wait on the async-results. Whenever a result is finished, you pop the next task from the tasks-deque and submit it into the pool. When all tasks are submitted, you just await the remaining async_results in a second loop.
Output from this example looks like this:
2018-11-20 20:21:55.430073: Initial distribution of 4 tasks.
2018-11-20 20:21:55.430166: Waiting for async_result.
150000000
2018-11-20 20:22:12.306330: Submitting next task.
2018-11-20 20:22:12.306414: Waiting for async_result.
150000002
150000003
150000001
2018-11-20 20:22:17.715737: Submitting next task.
2018-11-20 20:22:17.715876: Waiting for async_result.
2018-11-20 20:22:17.715922: Submitting next task.
2018-11-20 20:22:17.726139: Waiting for async_result.
2018-11-20 20:22:17.726201: Submitting next task.
2018-11-20 20:22:17.726263: Waiting for async_result.
150000004
2018-11-20 20:22:32.358946: Submitting next task.
2018-11-20 20:22:32.359040: Waiting for async_result.
150000007
150000005
2018-11-20 20:22:37.984947: Submitting next task.
2018-11-20 20:22:37.990142: All tasks submitted.
2018-11-20 20:22:37.990173: Waiting for async_result.
150000006
2018-11-20 20:22:38.783913: Waiting for async_result.
2018-11-20 20:22:38.783988: Waiting for async_result.
150000008
2018-11-20 20:22:46.364376: Waiting for async_result.
150000009
[150000000, 150000001, 150000002, 150000003, 150000004, 150000005, 150000006, 150000007, 150000008, 150000009]
Process finished with exit code 0
add a comment |
There's no build-in way if that's your question. A worker idling just blocks at task = get()
. get()
here is the corresponding method of either a multiprocessing.SimpleQueue
, in case your pool is a process-pool, or of a queue.Queue
, in case you use multiprocessing.dummy.Pool
aka multiprocessing.pool.ThreadPool
. There's no internal bookkeeping about the number of idling workers, it's just .get()
and .put()
on queues what triggers distribution of tasks and fetching of results.
You can however, get the count of outstanding tasks when you check the length of the internal pool._cache
. After a processed tasks returns from a worker, the entry (ApplyResult
-instance) there gets deleted. So when you evaluate max(pool._processes - len(pool._cache), 0)
you would get a number of idling workers. The actual value is not reliable, though. Pool
uses three threads for managing its internals...
I built an example which didn't break in testing, but I don't consider it safe to use, although the only danger here would be that a task might be submitted too early. It would also include some time.sleep()
to prevent busy-waiting, but that's always inefficient, because it doesn't (just) unblock as soon there is actually something to do.
Instead, if you insist on using multiprocessing.Pool
, you could do something like the example below shows. I'm using a collections.deque
here as a structure for tasks and results to append()
on the right end and popping from the left end. deque
is optimized for such operations and also used to implement queue.Queue
.
from datetime import datetime
from collections import deque
from multiprocessing import Pool
def busy_foo(x):
for _ in range(int(x)):
1 - 1
print(x)
return x
if __name__ == '__main__':
N_WORKERS = 4
tasks = deque(
[(busy_foo, (i,)) for i in range(int(150e6), int(150e6 + 10))]
)
pool = Pool(N_WORKERS)
print(f'{datetime.now()}: Initial distribution of {N_WORKERS} tasks.')
async_results = deque(
[pool.apply_async(*tasks.popleft()) for _ in range(N_WORKERS)]
)
fetched_results =
while tasks: # while still undistributed (non-popped) tasks left
print(f'{datetime.now()}: Waiting for async_result.')
# `.get()` blocks until result available
fetched_results.append(async_results.popleft().get())
print(f'{datetime.now()}: Submitting next task.')
async_results.append(pool.apply_async(*tasks.popleft()))
print(f'{datetime.now()}: All tasks submitted.')
while async_results: # get rest of outstanding results
print(f'{datetime.now()}: Waiting for async_result.')
fetched_results.append(async_results.popleft().get())
pool.close()
pool.join()
print('n', fetched_results)
What happens is that you, in a first round, distribute as much tasks as much workers you have in your pool. Then you while-loop over the remaining tasks and block-wait on the async-results. Whenever a result is finished, you pop the next task from the tasks-deque and submit it into the pool. When all tasks are submitted, you just await the remaining async_results in a second loop.
Output from this example looks like this:
2018-11-20 20:21:55.430073: Initial distribution of 4 tasks.
2018-11-20 20:21:55.430166: Waiting for async_result.
150000000
2018-11-20 20:22:12.306330: Submitting next task.
2018-11-20 20:22:12.306414: Waiting for async_result.
150000002
150000003
150000001
2018-11-20 20:22:17.715737: Submitting next task.
2018-11-20 20:22:17.715876: Waiting for async_result.
2018-11-20 20:22:17.715922: Submitting next task.
2018-11-20 20:22:17.726139: Waiting for async_result.
2018-11-20 20:22:17.726201: Submitting next task.
2018-11-20 20:22:17.726263: Waiting for async_result.
150000004
2018-11-20 20:22:32.358946: Submitting next task.
2018-11-20 20:22:32.359040: Waiting for async_result.
150000007
150000005
2018-11-20 20:22:37.984947: Submitting next task.
2018-11-20 20:22:37.990142: All tasks submitted.
2018-11-20 20:22:37.990173: Waiting for async_result.
150000006
2018-11-20 20:22:38.783913: Waiting for async_result.
2018-11-20 20:22:38.783988: Waiting for async_result.
150000008
2018-11-20 20:22:46.364376: Waiting for async_result.
150000009
[150000000, 150000001, 150000002, 150000003, 150000004, 150000005, 150000006, 150000007, 150000008, 150000009]
Process finished with exit code 0
There's no build-in way if that's your question. A worker idling just blocks at task = get()
. get()
here is the corresponding method of either a multiprocessing.SimpleQueue
, in case your pool is a process-pool, or of a queue.Queue
, in case you use multiprocessing.dummy.Pool
aka multiprocessing.pool.ThreadPool
. There's no internal bookkeeping about the number of idling workers, it's just .get()
and .put()
on queues what triggers distribution of tasks and fetching of results.
You can however, get the count of outstanding tasks when you check the length of the internal pool._cache
. After a processed tasks returns from a worker, the entry (ApplyResult
-instance) there gets deleted. So when you evaluate max(pool._processes - len(pool._cache), 0)
you would get a number of idling workers. The actual value is not reliable, though. Pool
uses three threads for managing its internals...
I built an example which didn't break in testing, but I don't consider it safe to use, although the only danger here would be that a task might be submitted too early. It would also include some time.sleep()
to prevent busy-waiting, but that's always inefficient, because it doesn't (just) unblock as soon there is actually something to do.
Instead, if you insist on using multiprocessing.Pool
, you could do something like the example below shows. I'm using a collections.deque
here as a structure for tasks and results to append()
on the right end and popping from the left end. deque
is optimized for such operations and also used to implement queue.Queue
.
from datetime import datetime
from collections import deque
from multiprocessing import Pool
def busy_foo(x):
for _ in range(int(x)):
1 - 1
print(x)
return x
if __name__ == '__main__':
N_WORKERS = 4
tasks = deque(
[(busy_foo, (i,)) for i in range(int(150e6), int(150e6 + 10))]
)
pool = Pool(N_WORKERS)
print(f'{datetime.now()}: Initial distribution of {N_WORKERS} tasks.')
async_results = deque(
[pool.apply_async(*tasks.popleft()) for _ in range(N_WORKERS)]
)
fetched_results =
while tasks: # while still undistributed (non-popped) tasks left
print(f'{datetime.now()}: Waiting for async_result.')
# `.get()` blocks until result available
fetched_results.append(async_results.popleft().get())
print(f'{datetime.now()}: Submitting next task.')
async_results.append(pool.apply_async(*tasks.popleft()))
print(f'{datetime.now()}: All tasks submitted.')
while async_results: # get rest of outstanding results
print(f'{datetime.now()}: Waiting for async_result.')
fetched_results.append(async_results.popleft().get())
pool.close()
pool.join()
print('n', fetched_results)
What happens is that you, in a first round, distribute as much tasks as much workers you have in your pool. Then you while-loop over the remaining tasks and block-wait on the async-results. Whenever a result is finished, you pop the next task from the tasks-deque and submit it into the pool. When all tasks are submitted, you just await the remaining async_results in a second loop.
Output from this example looks like this:
2018-11-20 20:21:55.430073: Initial distribution of 4 tasks.
2018-11-20 20:21:55.430166: Waiting for async_result.
150000000
2018-11-20 20:22:12.306330: Submitting next task.
2018-11-20 20:22:12.306414: Waiting for async_result.
150000002
150000003
150000001
2018-11-20 20:22:17.715737: Submitting next task.
2018-11-20 20:22:17.715876: Waiting for async_result.
2018-11-20 20:22:17.715922: Submitting next task.
2018-11-20 20:22:17.726139: Waiting for async_result.
2018-11-20 20:22:17.726201: Submitting next task.
2018-11-20 20:22:17.726263: Waiting for async_result.
150000004
2018-11-20 20:22:32.358946: Submitting next task.
2018-11-20 20:22:32.359040: Waiting for async_result.
150000007
150000005
2018-11-20 20:22:37.984947: Submitting next task.
2018-11-20 20:22:37.990142: All tasks submitted.
2018-11-20 20:22:37.990173: Waiting for async_result.
150000006
2018-11-20 20:22:38.783913: Waiting for async_result.
2018-11-20 20:22:38.783988: Waiting for async_result.
150000008
2018-11-20 20:22:46.364376: Waiting for async_result.
150000009
[150000000, 150000001, 150000002, 150000003, 150000004, 150000005, 150000006, 150000007, 150000008, 150000009]
Process finished with exit code 0
edited Nov 20 at 22:04
answered Nov 20 at 19:43


Darkonaut
2,5832718
2,5832718
add a comment |
add a comment |
Thanks for contributing an answer to Stack Overflow!
- Please be sure to answer the question. Provide details and share your research!
But avoid …
- Asking for help, clarification, or responding to other answers.
- Making statements based on opinion; back them up with references or personal experience.
To learn more, see our tips on writing great answers.
Some of your past answers have not been well-received, and you're in danger of being blocked from answering.
Please pay close attention to the following guidance:
- Please be sure to answer the question. Provide details and share your research!
But avoid …
- Asking for help, clarification, or responding to other answers.
- Making statements based on opinion; back them up with references or personal experience.
To learn more, see our tips on writing great answers.
Sign up or log in
StackExchange.ready(function () {
StackExchange.helpers.onClickDraftSave('#login-link');
});
Sign up using Google
Sign up using Facebook
Sign up using Email and Password
Post as a guest
Required, but never shown
StackExchange.ready(
function () {
StackExchange.openid.initPostLogin('.new-post-login', 'https%3a%2f%2fstackoverflow.com%2fquestions%2f53387493%2fhow-to-get-the-count-of-free-used-processes-threads-in-a-process-pool%23new-answer', 'question_page');
}
);
Post as a guest
Required, but never shown
Sign up or log in
StackExchange.ready(function () {
StackExchange.helpers.onClickDraftSave('#login-link');
});
Sign up using Google
Sign up using Facebook
Sign up using Email and Password
Post as a guest
Required, but never shown
Sign up or log in
StackExchange.ready(function () {
StackExchange.helpers.onClickDraftSave('#login-link');
});
Sign up using Google
Sign up using Facebook
Sign up using Email and Password
Post as a guest
Required, but never shown
Sign up or log in
StackExchange.ready(function () {
StackExchange.helpers.onClickDraftSave('#login-link');
});
Sign up using Google
Sign up using Facebook
Sign up using Email and Password
Sign up using Google
Sign up using Facebook
Sign up using Email and Password
Post as a guest
Required, but never shown
Required, but never shown
Required, but never shown
Required, but never shown
Required, but never shown
Required, but never shown
Required, but never shown
Required, but never shown
Required, but never shown
rE,uhYG9s8,oQw 6U1dzoep2LeBQS I5qD0